Opinion
100 deals and more than $7B in global funding in Q1 2021: 3 key differentiators of winning healthcare AI startups
Building successful AI startups in healthcare requires understanding the unique challenges of the technology. Healthcare founders and investors should acknowledge this ‘special recipe’.
These incredible applications were not possible in the previous generation of traditional software companies. Traditional software relies mostly on preprogrammed if/then rules. AI, in contrast, is able to identify patterns (many of them not observable or learnable by humans) on its own. This ability is critical as medical data is so vast that it is impossible to know all possible outcomes in advance (and thus write if/then statements that predict them).
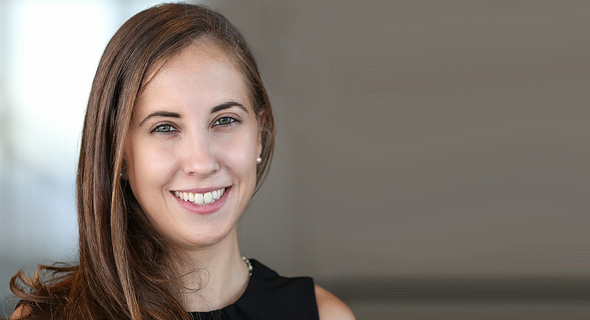
It’s no wonder, then, that venture capital funding for digital healthcare is on track for a record-breaking year in 2021 - with nearly 100 deals and more than $7 billion in global funding in Q1 alone. As investors at Pitango HealthTech, Pitango Venture Capital’s dedicated healthcare fund, we see dozens of AI startups with outstanding technology each year.
But outstanding technology alone doesn’t mean that an AI startup will be successful. AI technology creates complexities and challenges in startups that need to be acknowledged and overcome. Founders and investors need to understand these complexities, so they can set the right expectations and properly build companies.
Let’s look at three differentiators that can make (or break) healthcare AI startups.
1. Selecting use cases with the ‘Path of Least Resistance’
AI can provide incredible insights and analyses. But its outputs can’t always be traced and explained. This is a challenge to physicians or patients who need to know why a certain conclusion was reached.
Many successful AI startups started out by tackling simple use cases where explainability and traceability are less important--i.e. where it’s okay to not understand the “why” behind the model’s decision, and easier to implement the product in a user’s workflow.
Examples of this are back office administrative or billing tasks that are highly manual today. These use cases do not require regulatory approval, clinical validation, or deep physician buy-in as they do not intervene in the medical workflow. Olive AI, a U.S startup with solutions in supply chain, shared services, and finance, is one such successful example.
This does not mean it’s impossible to build successful companies based on clinical use cases--only that the burden of proof for the technology is higher, and that back office use cases can be good initial applications.
2. Budgeting appropriately
Unlike software, AI requires substantial ongoing investment in data work--both internally within the company, and on the customer side. Internally, AI startups need to troubleshoot, monitor, and constantly improve their models. They also pay significantly more for cloud and computing resources. In addition, since many healthcare customers do not have data science experts internally, startups need to provide the professional services and support teams to help them implement, maintain, read and make sense of the AI.
Therefore, successful healthcare startups will devote substantial resources to technical teams to improve their models and to help customers make use of the AI solution they provide. Qventus and LeanTaas, American companies using AI to find efficiencies in hospital operations, do this well and have consulting teams that augment their technology with hands-on professional services.
- Israel's most important “natural” resource
- To boldly lead the global space industry
- Should Israel be at the forefront when it comes to the adoption of digital currency solutions?
From a financial and company-building standpoint, this means that AI companies spend more relative to traditional SaaS software startups. Investors and founders must be cognizant of these expected lower margins, and take them as “part of the package” when building healthcare AI companies.
3. Creating a differentiated data strategy
AI training algorithms, which are used to create a product’s predictive model, need vast amounts of unique data. Obtaining such data is no easy feat.
A strong data strategy is, therefore, a critical success factor for a healthcare AI startup. One possible route is creating original proprietary data--for instance collecting patient-reported outcomes or biomarkers from sensors. In the absence of proprietary data, speed and scale are critical. Many startups may get access to Electronic Health Record data, but those that do so the quickest--either through a strong partnership or by spending a lot of money--will end up building better products and prevailing over their competition.
Truveta is a U.S example of this, Founded by 14 of the largest U.S health systems to create a single data marketplace, it has an invaluable data asset by definition and from the get-go that many other similar companies would find hard to compete with.
--
At Pitango VC, we know that treating AI as a pure technology play is not enough to succeed. AI becomes a defensible moat only when combined with additional key operational capabilities and differentiators. There are of course many other qualities for successful healthcare companies: a strong team, an understanding of the value-chain and stakeholders, and a solid go-to-market and pricing strategy, just to name a few. But the above three attributes are unique to those startups aiming to build successful AI companies. We are excited to see and meet such companies and teams, so don’t hesitate to reach out to us if you are building one.
Maya Perl is the Principal, Pitango HealthTech.