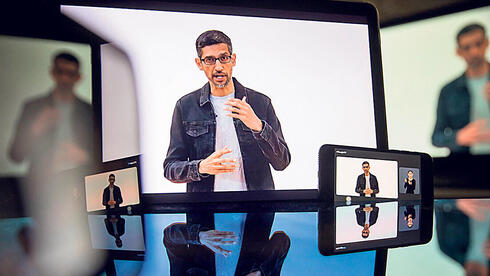
Lost in translation: GenAI’s mother tongue discrimination
Lost in translation: GenAI’s mother tongue discrimination
Artificial intelligence tends to translate and learn uncommon languages poorly. AI tools like ChatGPT train on inferior products in these languages, which will result in their billions of speakers suffering from technological delays and discrimination
Which source of information would you prefer to serve as a basis for the great discovery of the 21st century? Constitutive and complex texts or a pile of horribly translated garbage? According to research from the artificial intelligence lab of AWS (Amazon's cloud division), the second option is the current reality. A paper published this month found that more than half of the sentences on the Internet were translated from English into two or more languages at a low quality due to the use of machine translation (MT). This pile of inferior translations is also the body of knowledge of artificial intelligence models, in what seems to be dooming many of us to technological backwardness.
The researchers, who submitted their research to the platform arXiv, built a collection of 6.38 billion sentences harvested from the web. In this collection, the researchers examined groups of sentences that are direct translations of each other in three or more languages. This examination revealed that approximately 58% of the sentences in the collection had equivalents in at least three languages, or simply put, most of the Internet is simply translated. And the examination brought up another finding - most of the translation is simply bad.
How does that happen? In a way that seems almost counterintuitive, "high-resource" languages like French or English translate into fewer languages than "low-resource" languages. In practice, languages that have a low internet presence are the ones that are translated much more, and that low internet presence makes them victims of machine translation.
1. Short sentences, shallow topics
The languages with "high resources" are few. At the top of the list is English, followed by Chinese (Mandarin), Arabic and French. Then you can also add German, Portuguese, Spanish and Finnish. These languages have large and accessible collections of digital text and transcribed recorded speech. For the other 700 languages, resources are substantially low. In the study it was found that content in languages with high resources is in the bottom tenth of the number of translations, with translation into three additional languages on average; Whereas content in languages with low resources such as Xhosa (which is spoken in South Africa and Zimbabwe, and in total by about 20 million people) has an average translation into another 7.6 languages.
The problem does not end here. In languages that have many translations, a selection bias towards short and predictable sentences of between five and ten words was also found. These are low quality texts that require very little expertise or knowledge to create and the topics are also generic such as "six tips for boat owners", or "the decision to be happy". This bias of mass translation of low-quality texts, the study claims, stems from the translators' desire to produce multiple contents that will generate revenue from ads. This means, the study concludes, that much of the content on the Internet is bad machine translation of low-resource languages. "As a sentence has been translated into more languages, the quality of its translations is lower, indicating a higher prevalence of machine translation," the researchers wrote.
Thus we got a pincer movement that corrupts languages for generations, a kind of strange situation where a potential "death spiral" seems almost inevitable: companies like Microsoft and Google use replica data on the Internet to train their models, and low-resource languages are not well represented on the Internet. Therefore, there is less data to train the specific models for these languages, but they deploy tools based on these poorly trained models.
The same models are used as aids to machine translation tools, and the same websites deploy machine translation to translate content into languages other than English. The effect continues to spread as the artificial intelligence tools continue to train on this data that they eventually had a hand in translating as well. This dynamic can trap the models and content sites in feedback loops that will propagate low-resource languages in their lowest and worst form.
This means that language models in languages with low resources will be significantly worse and there will be a cumulative effect of technological backwardness for products based on such languages. "Modern artificial intelligence is made possible with the help of huge amounts of training data," the researchers note. "Training on this scale is only possible with data collected from the Internet. Our findings raise many concerns for multilingual model builders, who may build models that are less fluent and more mistake prone."
2. ChatGPT for English speakers only
This research echoes what users of low-resource languages have long known. Since ChatGPT and its alternatives from Google and others were launched, they thrive in a handful of languages such as English, French, German and also Chinese, but fail resoundingly in many other languages such as Swahili, Bengali, Urdu or Thai for the hundreds of millions who master them. This is a failure at the lowest levels required of chats, which includes an inability to handle the simplest of tasks.
Salvation will not come from the tech giants leading the artificial intelligence race. They recognize the language problem, and still treat English as the most important language - sort of the default. All the techniques that are developed in this context are developed specifically for the English language (and similar developments are also made in Chinese in China).
Those who can fix the problem are mainly local startups from Africa and Southeast Asia, which have begun to employ expert content writers in the local languages, whose task is to produce quality texts in those languages with low resources and to rate with the help of experts translations made by a machine - what is known as "reinforcement learning from human feedback".
According to a report by "The Washington Post" from August, these experts receive a fifth of the payment of their counterparts in languages with high resources such as French and German, who are also employed in similar projects to strengthen the models.
Alongside them is also one American startup, Scale AI, which usually pays contractor workers in many countries around the world for micro-tasks related to training models. This startup's service is used by Meta, Google and OpenAI.
The International Monetary Fund recently estimated that artificial intelligence will deepen inequality in the world and affect 40% of jobs. The IMF notes that the impact of artificial intelligence is not only on jobs. While the "artificial intelligence revolution" is aggressively pushed by the private market and products based on language models are pushed to deeply integrate with all elements of life—from work to all social services—those who speak unrepresented languages will suffer multiple technological delays simply because of the little emphasis that can be placed on perfecting the models to suit their language.
The IMF is wrong to cite technology as a reason for deepening inequality. It is not the artificial intelligence that is responsible for this, but the person who develops and supervises it. If all concerned continue to bury their heads in an English, French and German based reality, everyone else will be left behind.