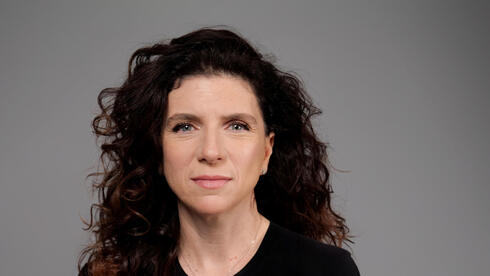
Opinion
The AI revolution: From private use to business growth
With the global AI market projected to reach $ 1.5 trillion by 2030, the opportunities for business AI are endless. However, according to Orna Kleinmann, CEO of SAP's R&D Center in Israel, to turn these opportunities into tangible outcomes, businesses must select the right tools and partners, ensure the chosen model is trustworthy, reliable, and unbiased, and be prepared to go the extra mile.
Artificial Intelligence, AKA "AI," is no longer just a buzzword; it has become an integral part of daily life, often without even realizing it. The turning point for any innovative technology occurs when it transitions from mere "hype" into real-world application.
If 2023 marked the year consumers gained access to AI, 2024 will see its integration into business management, making it critical for operational efficiency. Like any successful consumer product, businesses will naturally seek to harness AI's potential for their competitive advantage.
However, there is a fundamental difference between the implementation of AI tools by individuals vs. the business world, primarily due to the potential risks and costs associated with bias, errors, and "AI hallucinations" (where an AI model generates false or misleading outputs that seem credible). Although appropriate and continuous training of the AI model should reduce such phenomena, biases and errors remain prevalent and frequently encountered by users. For instance, when I recently checked for gender bias, I used a text-to-image (AI Large Language Model - LLM) designed to generate images from verbal descriptions, requesting an image of surgeons in the operating room. Since English lacks gender-specific nouns like Hebrew or other languages, I obtained several results that exclusively depicted male surgeons, most of whom were white. Alternatively, when I asked for images of nurses, most images featured women, some with ethnic diversity. A similar pattern emerged when I requested an image of a software developer – the results only portrayed young males, which starkly contrasts the gender and age diversity found in our development center, as well as in other startups, multinational companies, and the broader tech ecosystem in Israel and worldwide.
When used by individuals, the impact of such biases may be frustrating and misleading, but it is relatively insignificant. However, the stakes are far higher in business applications, where such biases can lead to anything from revenue loss and damaged reputation to tangible injury to customers or even society at large.
Hence, the following key questions arise: What is the current maturity level of AI technologies for business purposes? How can privacy, particularly critical business information, be safeguarded?
With regard to maturity, it is essential, first of all, to explain what business AI actually is: Business AI is the way we enable large companies, who have their own data, to run AI on their data by implementing models that are trained on this type of data to begin with. In other words, they seek to improve themselves and their processes, especially vis-a-vis their customers. For example, business AI may ask, "How can I generate more sales in a given geographic area within the United States?" and then offer a response based on the company's own data or the same software trained with Data of dozens of companies of similar features. That is, in business AI, the business feeds its internal information into the AI model and receives an AI-based business application that allows it to send a question and receive an answer, thereby generating tools for effective business management and improving the insights obtained from the vast amount of data accumulated in the organization over the years.
However, the business sector comprises companies that differ from one another in various parameters, and it is difficult, if not impossible, to find a single AI solution that will suit them all. Therefore, managers need to handpick the right partners and technology suitable for their needs when seeking to integrate AI into the business. Today, several AI solutions are available on the market that companies can adopt without the need to develop their own from scratch. Only a few businesses have the resources to create a custom AI model. Still, for those who cannot, the current market offerings are undoubtedly mature enough to provide businesses with a competitive advantage. Furthermore, most companies rely on a range of existing, frequently legacy systems that have been in place for years. Subsequently, there is a need to "inject" AI capabilities into these systems without compromising sensitive data, run models and manipulate the information in-house. Companies typically refrain from exporting data from their ERP or CRM systems to be externally manipulated due to data security, data integrity, and other factors.
When it comes to privacy, businesses must avoid developing biased AI models and ensure that data remains securely within the organization. Proper collection and storage of business data are essential for processing by AI models, but equally important is the seamless integration between various business systems. Therefore, ensuring AI's success in the business landscape requires adopting a unified data strategy. The alignment of data across various sources within an organization provides a solid foundation for accurate AI training and deployment. A unified approach to data management preserves context, ensuring that the AI model operates with a full understanding of the data's origins, purpose, and connections. This comprehensive view enhances AI’s ability to deliver actionable insights, optimize decision-making, and drive business efficiency. Moreover, by maintaining data coherence and integrity, businesses can mitigate risks associated with flawed AI outputs and create a reliable, single source of truth, ensuring the data is relevant (in both quality and quantity), reliable (the model's accuracy level), and trustworthy (free from false information and biases). Without such a strategy, even the most advanced AI tools are likely to fall short of their potential, ultimately leading to missed opportunities and operational inefficiencies.
Integrating business AI would undoubtedly deliver a strong return on investment and even boost a company's bottom line. The potential benefits of AI applications are vast: from automating routine tasks to free up human resources for more strategic tasks to analyzing data for trend identification and enhancing customer loyalty and experience through personalized recommendations, among other things. However, businesses must remain pragmatic about the potential to generate real added value from each AI application.
Companies that successfully implemented business AI tools are already yielding impressive results. For example, American Sugar Refining, an American company that manufactures sugar cane-based products, uses artificial intelligence to predict its transportation costs more accurately. Predictions that once took a fair amount of human time are now generated automatically within seconds. The company generated 2,200 forecasts based on 6-months of data and reached a 95% accuracy level.
Motor Oil Group, a Greek energy company, has adopted AI tools to monitor the health of its refinery equipment to minimize downtime and reduce maintenance costs. It created a model for predicting adverse events based on sensor data and fed them into user-friendly dashboards, which in turn generated email alerts. Thus, the model could explain adverse events with a 77% accuracy level and predict future sensor measurements with a 70% accuracy level.
While the potential of business AI is vast, effective implementation still poses a challenge for many companies. According to the extensive DLA Piper Law Firm study conducted among 600 global business leaders, nearly half of the companies surveyed had to halt or slow down AI-based projects. The research highlights a significant gap in understanding how to implement AI effectively to drive growth and generate added value for customers. The key to successfully leveraging AI for improved business performance lies in selecting the right technology and partners for the journey.
And one last point that must be emphasized: As with any learning process, integrating AI tools within an organization warrants persistence. It is imperative to remain task-oriented and not be discouraged if immediate results are elusive. Trial and error, patience, and a commitment to learning across all levels of the organization are essential to unlocking AI's true value.
With the global AI market projected to reach US$ 1.5 trillion by 2030, the opportunities for business AI are endless. However, to turn these opportunities into tangible outcomes, businesses must select the right tools and partners, ensure the chosen model is trustworthy, reliable, and unbiased, and be prepared to go the extra mile.
Orna Kleinmann is CEO of SAP's R&D Center in Israel.