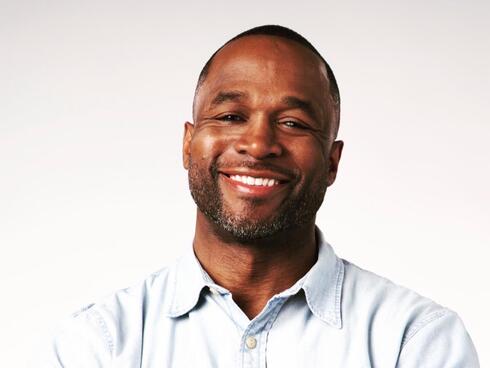
OpinionHow AI is transforming climate tech and reshaping the future of sustainability
Opinion
How AI is transforming climate tech and reshaping the future of sustainability
From wildfire prevention to renewable energy optimization, AI is tackling some of the biggest climate challenges, writes Dion Harris, Director of Accelerated Computing at NVIDIA.
There is an exciting potential for applying artificial intelligence (AI) in climate technology. AI can be used to address various climate challenges, including improving weather forecasting, monitoring deforestation, optimizing farming practices, preventing wildfires, and promoting renewable energy. The obstacles to AI adoption in climate tech include data silos, lack of high-resolution data, and the need for powerful computing resources. There are efforts to overcome these challenges through digital twin technology, accelerated computing, and hybrid AI models. These solutions are leading to more accurate climate simulations and allowing for more effective climate action.
Key Areas of AI Impact in Climate Tech
Climate and Weather Modeling
AI can be used to accelerate climate and weather models, which can improve the speed, accuracy, and efficiency of these models. This can be helpful for industries such as agriculture, logistics, and disaster recovery. AI and digital twins can also be used to simulate weather systems and other climate-related phenomena, which can provide more accurate forecasts and planning tools.
Geospatial Monitoring and Detection
AI can be used for image classification and detection in environmental monitoring. For example, AI can help detect illegal deforestation in the Amazon by identifying new road formations, enabling early interventions, and reducing illegal deforestation.
Agritech and Sustainable Farming
AI can be used to optimize conditions such as temperature, humidity, and energy use in indoor farming and bug farming, which can ensure sustainable production. AI can also be used to improve resource management in agriculture, such as water, energy, and land, making farming more sustainable and efficient. Finally, AI can be used for optimizing genetic discovery and development activities for new biopesticide development.
Wildfire Detection and Prevention
AI, combined with geospatial and ground-based technologies, can be used to predict and prevent wildfires.
Renewable Energy
AI can help optimize energy production, manage grids, and improve efficiency in renewable energy sources like solar and wind.
Broader Sustainable Technologies
AI can be broadly applied across different climate tech sectors, including start-ups and large enterprises, to create sustainable technologies in agriculture, weather systems, energy, and environmental monitoring.
Challenges of Implementing AI in Climate Tech
Climate data is fragmented, coming from sources like satellites and historical datasets (e.g., ERA5), making it hard to centralize for AI training. Digital twin technology helps address these data silos by integrating various datasets into a federated model, providing a unified view of the climate system. Projects like Earth 2 and Project Destiny aim to aggregate this data for easier AI and simulation use.
High-resolution datasets are crucial for accurate AI climate models, but large-scale simulations are required to produce them. Companies like NVIDIA use GPU-optimized models (e.g., WARF, ICON, IMPASSE) and platforms like Grace Hopper to accelerate simulations, creating kilometer-scale, high-fidelity data efficiently.
AI models depend on data from complex and computationally intensive numerical weather models, which NVIDIA has accelerated using GPU-based systems. This allows these models to run faster, generating the high-resolution data needed for AI training and improving weather prediction accuracy.
The Earth’s climate system is complex, involving multiple interacting components like the ocean and atmosphere. Digital twins and data federation models, will be one of the topics on which we will further elaborate during NVIDIA's GTC 2025, which will take place between 17-20th of march, integrate diverse datasets into a unified system, enabling more accurate AI-driven climate simulations by capturing these interrelations.
Predicting long-term climate changes is challenging due to limited historical data. A hybrid AI approach, combining data-driven and physics-based models, addresses this by incorporating physical laws like thermodynamics, making predictions more accurate even with incomplete datasets.
High-resolution climate models require supercomputing resources that are often inaccessible, especially in developing countries. Platforms like NVIDIA’s Omniverse help democratize access to climate data and models, enabling collaboration and sharing, even among organizations with fewer resources.
Validating AI climate models is difficult due to changing conditions. Hindcasting—using historical data to test models’ accuracy—helps gauge prediction reliability by comparing results with known outcomes.
Many climate datasets are low-resolution, limiting actionable insights, especially for disaster preparedness in vulnerable regions. AI-based techniques, like those used by platforms like Corrdiff, enhance dataset resolution, enabling local governments to make more informed decisions.
Dion Harris is the Director of Accelerated Computing at NVIDIA.