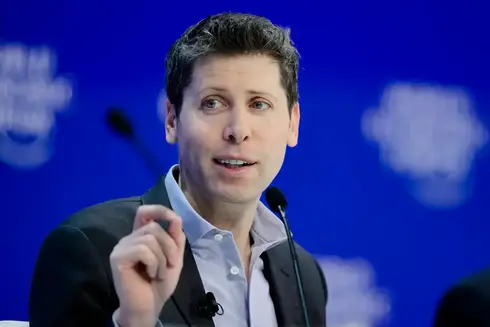
The hidden battle for data dominance: AI giants' secret struggle
The hidden battle for data dominance: AI giants' secret struggle
The generative artificial intelligence market is engaged in a new competitive race for a particularly critical resource: data. According to estimates, soon the data available online will not keep up with the required pace and there will be nothing to train the models behind services like ChatGTP and Gemini. The obvious solutions: transcribing videos and recordings or a technological development that circumvents the problem
The generative artificial intelligence (GenAI) market is currently in the midst of a clandestine competition. It's not merely about capturing market share or establishing dominance, as is typical in new markets. Nor is it solely about the intense competition for computing power, given the limited supply of high-performance chips. Rather, it's a race for one of the critical resources for developing advanced models: data. Despite the seemingly endless supply of content in today's digital age, the existing amount of content on the web will not suffice to satisfy the hunger of large AI models for long. Consequently, various companies in the field are determined to do whatever it takes to be the first to access the untapped reservoir of data
Is this the end of development?
There's a 90% chance that by 2028, the demand for high-quality textual data will outstrip supply.
Large language models (LLMs), such as OpenAI's ChatGTP and Google's Gemini, have achieved remarkable results thanks to diverse factors, including breakthroughs in algorithm development and advanced computing capabilities enabled by high-performance chips. Access to a vast amount of textual and other materials available online has also played a significant role in their success.
This approach is facilitated by the internet, which has evolved into the largest repository of human knowledge in history. The supply of content it provides for these models is growing exponentially day by day. Models draw strength from a variety of online data sources, including scientific articles, news stories, Wikipedia entries, social media posts, and digitized books. Each piece of data is broken down and fed to the model in small units called tokens. For example, OpenAI's GPT-4 is estimated to have been trained on 12 trillion tokens.
However, it's possible that even the seemingly inexhaustible online selection may not be enough to train new and more advanced models. For instance, GPT-5 is projected to require 60 trillion to 100 trillion tokens. Despite efforts to harness all available high-quality written and visual data on the web, a significant gap of between 10 trillion and 20 trillion tokens or more may remain. Experts estimate a 90% chance that by 2028, the demand for high-quality textual data will exceed the supply, significantly slowing down advancements in artificial intelligence.
Secret endeavors
Pursuing untapped data sources and novel training methods
In response, AI companies are actively seeking untapped sources of data and exploring new methods to train models. Ari Morcos, founder of DatologyAI and a former employee of Meta and Google's DeepMind, describes the lack of data as a pioneering problem with no acceptable solution.
For example, OpenAI is exploring the transcription of high-quality videos and audio recordings, including public YouTube videos, to train GPT-5. While some companies are experimenting with creating synthetic training materials using AI systems, researchers caution that this approach may produce incoherent data. All of these efforts are conducted in secret, as executives believe they can gain a competitive advantage.
This aspect of competition among companies parallels the race between European powers during the colonial period to claim ownership of unknown territories and exploit their resources. The transformation of superpowers into technology companies and the shift from gold and minerals to data beautifully illustrates the societal changes of recent centuries.
Creative solutions
Maximizing data utilization and reducing costs
Some companies are pursuing creative ways to maximize the use of existing data. DatologyAI has developed a method called curriculum learning, where data is fed to the model in a specific order to create smarter connections. This method achieves performance similar to traditional learning methods but with half the amount of data, significantly reducing training and operating costs for GenAI models.
Additionally, companies like OpenAI are experimenting with building smaller models tailored for individual tasks. According to Sam Altman, OpenAI's founder and CEO, the era of huge models may be coming to an end, with a focus on alternative improvements.
While the lack of sufficient data could potentially cause significant damage to the development of new models and have adverse effects on the field and the economy, experts believe this scenario is unlikely. They compare it to concerns about "peak oil" at the beginning of this century, which have been disproven with advancements in production technologies and shifts in demand toward sustainable energies. Similar developments may be seen in the field of AI, although the biggest uncertainty lies in anticipating breakthroughs.